Beyond One AI: How Multi-Agent Systems Are the Key to Complex Business Automation
- ϳԹվMay 6, 2025
- Last Modified onMay 6, 2025
Complexity is the norm in the business world. Whether you are dealing with a multi-tiered supply chain, intricate customer support systems, or fast-paced financial transactions, there's no shortage of tasks that require swift, coordinated action. While AI-powered agents are a key tool in automating many of these tasks, relying on a single agent isn't nearly enough. What businesses need is multi-agent systems that can communicate, share data, and adjust in real time.

What are Multi-Agent Systems in AI?
A multi-agent system (MAS) is a framework for managing complex tasks. In essence, it's a system made up of several autonomous AI agents that operate in parallel, each specializing in a particular function but working towards a common goal. Think of a team of experts, where each agent is an expert in a specific area:
- One agent might focus on inventory management.
- Another could be responsible for shipping logistics.
- A third might specialize in demand forecasting.
Each agent is equipped to make decisions independently, but they don't work in isolation. They share information and adjust their actions based on what's happening across the entire system. This dynamic, collaborative setup allows businesses to handle a range of tasks simultaneously while staying agile in the face of rapid changes.
Key Benefits of Multi-Agent Systems in Business
- Overcome Context Limitations: Each agent processes a specific subset of information, bypassing the token limits faced by single large models. This allows for deeper analysis of lengthy documents like financial reports or contracts.
- Modular Task Allocation: Agents are assigned focused roles such as data extraction, validation, or summarization, ensuring that each part of the workflow is handled by a component purpose-built for that function.
- Greater Transparency & Traceability: The modular design allows teams to audit the logic step by step. If errors occur, it's easier to pinpoint which agent or process introduced the issue.
- Faster Debugging and Issue Resolution: Teams can diagnose and fix problems more efficiently by targeting specific agents instead of retraining or troubleshooting an entire monolithic model.
- Targeted System Optimization: Improvements like refining an agent's logic or adding a human review step, can be made to individual components without disrupting the full pipeline.
- Flexible and Scalable Architecture: As businesses evolve, new agents can be introduced or existing ones adapted. The system can easily scale horizontally, handling additional complexity without performance trade-offs.
- Cross-Model Integration: Different agents can use different models optimized for their specific task (e.g., classification vs summarization), allowing for more precise and efficient execution across workflows.
How Industries are Putting Multi-Agent AI Systems to Work
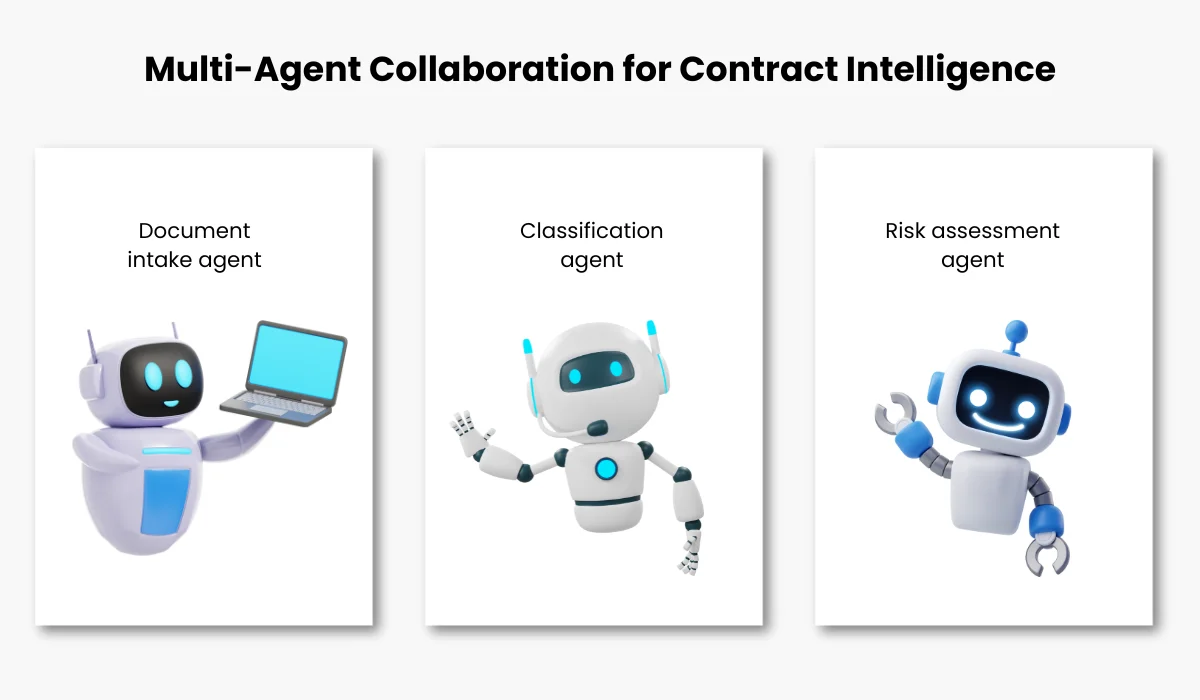
1. Legal Services
In the legal industry, multi-agent systems are transforming how law firms and corporate legal teams approach contract review. Instead of relying on paralegals to sift through pages manually, these firms deploy a chain of collaborative agents. One agent processes incoming agreements and structures the content. Another identifies and labels key clauses such as indemnity or termination.
A third compares those clauses against company-approved templates to catch deviations. Risk-focused agents evaluate potential exposure buried in the language, while summary agents package the findings into digestible briefs for final review. This structure doesn't just cut the time it takes to review contracts by more than half and raises the quality of oversight, surfacing risks that human reviewers might miss after hours of reading.
2. Financial Services
In financial services, where time-sensitive decisions are made based on vast volumes of market intelligence, multi-agent systems are helping analysts process data more comprehensively. Where one set of agents works on extracting financial metrics from various reports and statements, others handle the unstructured parts and translate them into actionable insights.
Specialized agents identify macro and micro trends from these inputs while reporting agents compile everything into charts, forecasts, and narrative summaries. This collaborative architecture allows firms to scale their research capabilities and act on insights that would otherwise take hours or days to uncover using manual tools.
3. Insurance
Insurance is another industry seeing rapid adoption. Historically, claims processing is a slow and error-prone function that becomes faster and more accurate with multi-agent automation. Agents digitize and interpret handwritten forms and PDFs, pull out relevant policy and claim details, verify coverage, and flag anomalies that might indicate fraud. From intake to payout calculation, each stage is handled by a specialized agent working in sequence, creating a claims pipeline that is both transparent and traceable. The result is faster settlements, fewer false approvals, and lower processing costs.
4. Real Estate
Even in a field known for its heavy paperwork and fragmented systems, multi-agent AI can simplify tasks like lease management. As properties scale across geographies and owners, agents are deployed to interpret lease documents, calculate escalations or rent cycles, verify compliance requirements, and track critical dates like renewals or terminations. This setup ensures teams stay ahead of obligations without manually monitoring hundreds of agreements, helping reduce legal risk and administrative drag.
5. Logistics and Supply Chain
Supply chain management is one of the most complex industries, with many moving parts: inventory management, supplier relationships, and shipping logistics, to name a few. Using a multi-agent system, companies can streamline operations by assigning agents to monitor different aspects of the supply chain. These agents can exchange real-time information and adapt swiftly to shifts such as changing demand or delivery disruptions.
For example, if one agent detects a delay at a warehouse, another can adjust delivery schedules, while a third adjusts inventory orders to maintain stock levels. This constant flow of communication helps maintain system efficiency, even when unexpected events arise.
6. Customer Support
In customer service, quick responses and precision are crucial. Multi-agent AI systems are ideal here as well. Instead of relying on a single AI chatbot that can only respond to a limited set of queries, different agents can handle different aspects of customer service. One might handle billing questions, another addresses technical support, and another can assist with product recommendations.
The beauty of this approach is that agents can communicate with one another and escalate issues when necessary, creating a seamless experience for customers. It also enables personalized service at scale.
These use cases all point to a common theme: multi-agent AI isn't just a technical novelty. It's a practical, structured solution to real-world business inefficiencies, one that improves decision accuracy while freeing professionals to focus on higher-order thinking.
Challenges and Considerations in Implementing a Multi-Agent AI System
Developing a multi-agent system isn't without its challenges, especially for businesses new to the concept.
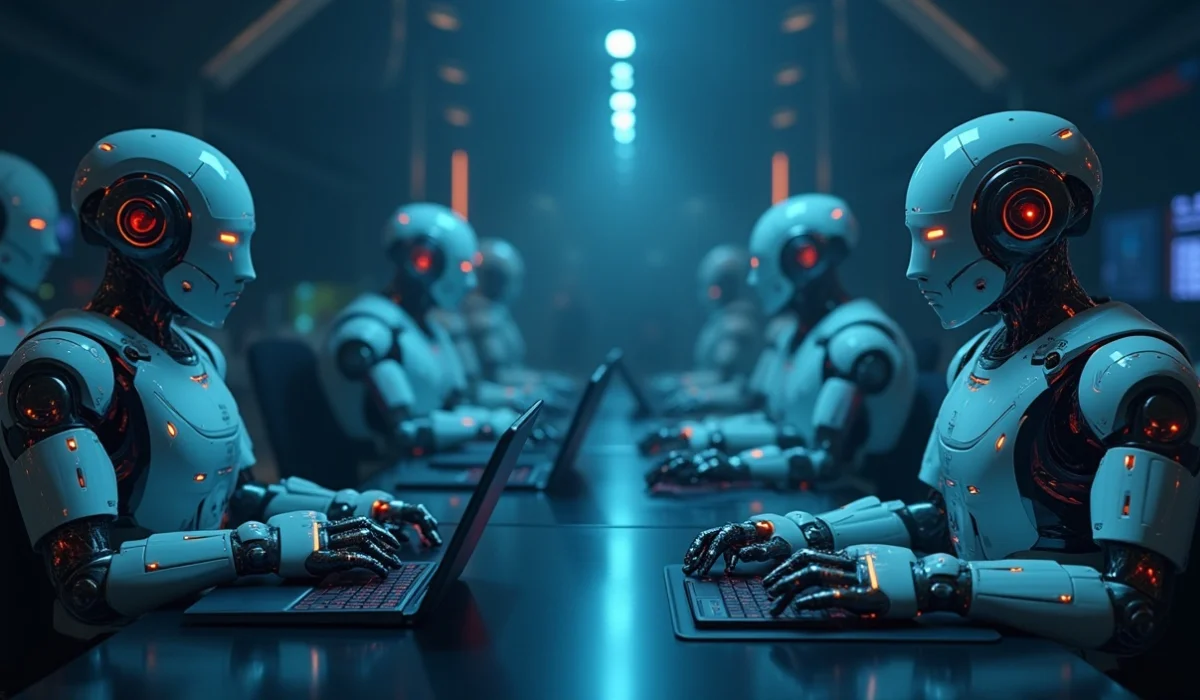
1. Complex Coordination
Ensuring they coordinate effectively with multiple agents working in parallel can be tricky. If not properly managed, agents can work at cross purposes, leading to inefficiencies or conflicting actions. This is where a well-defined communication protocol becomes essential, ensuring that agents understand their roles and how to interact.
2. Security Risks
Since MAS involves multiple agents communicating over networks, security is a top concern. Sensitive business data must be kept secure, and each agent must be protected from attacks. Implementing strong security measures, like encryption and authentication, is crucial to prevent data breaches.
3. Integration with Existing Systems
For many businesses, the challenge isn't just implementing a multi-agent system, it's integrating it with existing infrastructure. Whether it's legacy systems or third-party tools, seamless integration is key to ensuring the new system works without disrupting existing operations.
Conclusion
Businesses today face a new set of challenges that traditional, single-agent automation systems can't handle. Whether it's complexity, the need for real-time adaptability, or the demand for scalability, multi-agent collaboration offers a powerful solution. Businesses can streamline operations, improve efficiency, and scale as needed by breaking tasks into specialized, collaborative agents.
If you are trying to weigh the potential of multi-agent systems, ask yourself this: Can your current automation system scale, adapt in real time, and handle the increasing complexity of your business? If not, then multi-agent collaboration might just be the future you must invest in.
Liked what you read?
Subscribe to our newsletter